The development of new materials is full of challenges: It often takes years and requires sophisticated methods and testing. Artificial intelligence could change that.
Machine learning is a branch of AI. It has already been used successfully in material development at Freudenberg Technology Innovation (FTI). A broad-based team of about 10 Freudenberg experts is using the new methods to delve into materials-related questions from the Business Groups.
Several Freudenberg Group strategic projects are now dealing with the implementation of other AI- and simulation-supported methods. The goal: to accelerate the development of materials and optimize their characteristics profile for future use. This cuts costs and shrinks ecological footprints.
What happens to a seal’s hardness and its damping characteristics if the recipe for a product is changed? How can a material improve frictional behavior? What other raw material helps to increase a product’s durability?
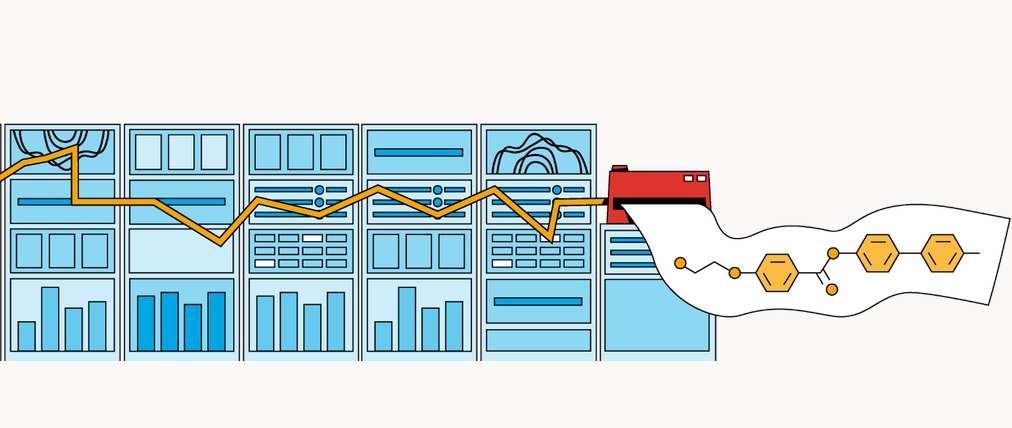
Machine learning programs save time and cut down on routine work.
Ansgar Komp, Director, Fundamental Sciences (FTI)
It once took several years of work and hundreds of lab tests and evaluations to answer these questions. “The process is faster and more efficient with machine learning. It saves time and cuts down on routine work,” said Ansgar Komp, Director, Fundamental Sciences at FTI. “First of all, the quality and availability of data is crucial. That means we capture the relevant lab data in a structured way. Then we apply the modern mathematical methods of artificial intelligence, which generate good proposals with just a few test series.” Experts with wide-ranging competencies in the simulation of materials and product characteristics help to ensure there are fewer “surprises” during the testing of new components. That has made it possible to develop the new Quantix Ultra class of materials efficiently, for example, Komp said.
One typical challenge in material development has been the limited availability of key materials during the pandemic. “That means that a good recipe has to be produced with another raw material without affecting its material characteristics,” he added. “Here the use of machine learning is helpful and makes a lot of sense. It is a way to achieve the goal more quickly and with less waste.”
Freudenberg forges ahead with AI methods
The Freudenberg group is expanding the use of AI-and simulation supported methods strategically. One example: The goal of the “Material Informatics” project is to make data-driven material development more efficient. Here the focus is on the development of elastomers, polymers and formulas for use in high-growth fields such as fuel cells. This requires the use of technologies that are fed test results from new characterization methods. “In this way, we can predict how a material functions, how it behaves,” said Dr. Harald Ehrentraut, Scientific Director, Fluid Dynamics at FTI. “These assessments from beyond our empirical world move us forward. We are encountering new material combinations that we never considered.”
When we use data elements as a source of information, it takes time and effort to make them available and structure them.
Dr. Corinna Hehlmann, Head of Data Analytics (FTI)
Another project, “Advanced Data Management,” deals with aspects of systematic and structured value creation using data. “In any project that uses data elements as sources of information, it takes time and effort to make them available and structure them,” said Dr. Corinna Hehlmann, Head of Data Analytics. “High-level data management accelerates this step.” Simulation methods also make it easier for product developers to forecast characteristics easier. In the “Macroscopic Simulation” project, component characteristics such as frictional behavior are explored with regard to operating conditions and the materials used. The characteristics are understood all the way from the atomic level to the component.
Development of new thinking
“We are working to turn the classic approach to development on its head. Instead of working painstakingly step-by-step toward the desired characteristics with test series, we will specify the characteristics profile and then propose materials with the help of simulations and AI methods. The best proposals will still be produced and investigated conventionally in the lab,” Komp said. “The advantage is that the entire development chain becomes more efficient.”
Artificial intelligence is a branch of information science. It imitates human cognitive capabilities by identifying and organizing information emerging from data inputs. Artificial intelligence can be based on programmed processes or generated with machine learning. The availability of large volumes of data and high-level computing power are required for these complex calculations. In the machine-learning process, an algorithm learns to perform a task independently through repetition.